Pipeline leak diagnosis using wavelet feature extraction and rad
نوشته شده به وسیله ی ali در تاریخ 95/12/30:: 5:44 صبح
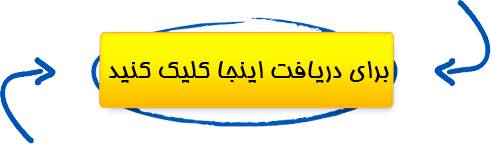
Pipeline leak diagnosis using wavelet feature extraction and radial basis function neural network (RBFدرNN) classifier دارای 13 صفحه می باشد و دارای تنظیمات در microsoft word می باشد و آماده پرینت یا چاپ است
فایل ورد Pipeline leak diagnosis using wavelet feature extraction and radial basis function neural network (RBFدرNN) classifier کاملا فرمت بندی و تنظیم شده در استاندارد دانشگاه و مراکز دولتی می باشد.
توجه : در صورت مشاهده بهم ریختگی احتمالی در متون زیر ،دلیل ان کپی کردن این مطالب از داخل فایل ورد می باشد و در فایل اصلی Pipeline leak diagnosis using wavelet feature extraction and radial basis function neural network (RBFدرNN) classifier،به هیچ وجه بهم ریختگی وجود ندارد
بخشی از متن Pipeline leak diagnosis using wavelet feature extraction and radial basis function neural network (RBFدرNN) classifier :
سال انتشار : 1394
نام کنفرانس یا همایش : پنجمین کنفرانس بین المللی رویکردهای نوین در نگهداشت انرژی
تعداد صفحات : 13
چکیده مقاله:
Transporting oil and gas through pipelines has a long history. Leaks, which are the main faults of pipelines, can cause serious problems such as safety, environmental and economic problems. Specially, in places where the pipeline crosses fresh water sources, for instance, rivers these affections are more noticeable and dangerous. Therefore, many methods and techniques for leak detection with various applications have been proposed to prevent further loss and danger. However, a good leak detection method not only decreases the false alarm rates, but also identifies the leak location and leak size. In this paper a novel leak detection approach is introduced, in which the leak detection problem is treated as a classification problem. Since in most practical cases, the end pressure of the pipeline has been fixed by equipment this novel approach only uses inlet pressure. By using OLGA software to simulate the pipeline different leakage conditions to monitor the changes of the pipeline inlet pressure in these conditions. In order to approximate these inlet pressure signals to practical conditions a normal noise is added. The wavelet domain features are extracted from the noisy signals and are adjusted as input stes for the radial basis function neural network (RBF-NN) classifier. The obtained results show a better performance compared to a RBF-NN classifier which uses statistical feature extraction techniques, instead
دانلود این فایل
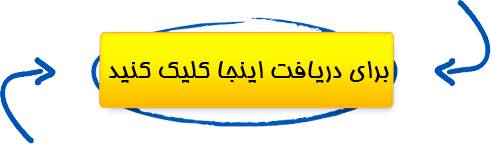
کلمات کلیدی :